Svetlana Borovkova: What is the value of alternative data for investment?
Svetlana Borovkova: What is the value of alternative data for investment?
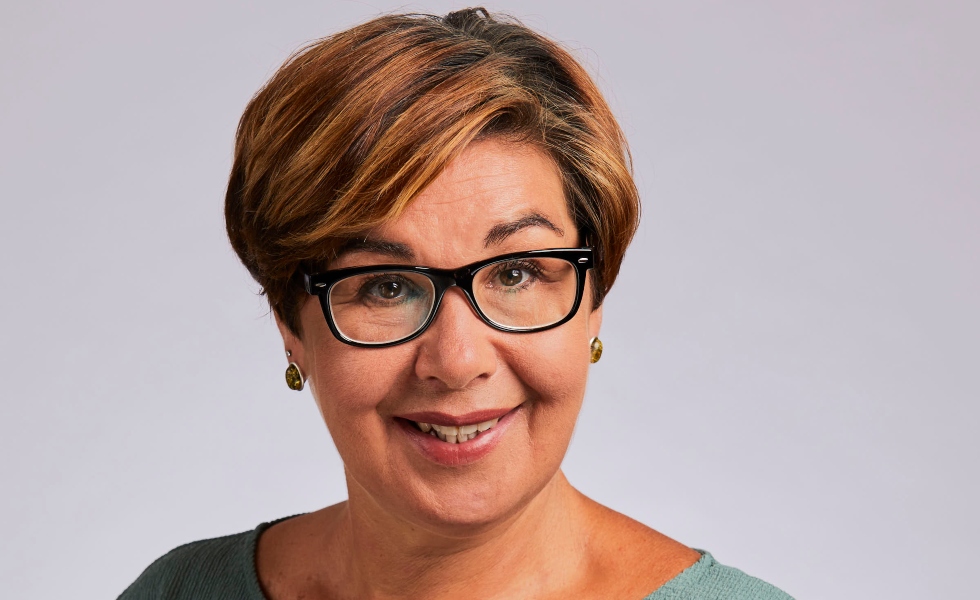
By Svetlana Borovkova, Head of Quant Modelling at Probability & Partners
‘Data is the new oil’: this catchy phrase summarizes the fact that data is becoming the world's most valuable commodity. The buzz around alternative data is tangible in the investment community as information retrieved from non-traditional sources is increasingly used as an input for investment decisions.
Alternative data can come from ingenuous new sources such as sensors, GPS locations or satellites. Other examples of alternative data sources are social media platforms, news wires, online spending and payment patterns of consumers.
Sources of alternative data
An interesting source of alternative data is satellite imagery, which can be applied to investments in numerous ways. For example, one can monitor the number of cars in parking lots of retailers to forecast their revenue, or the number of ships passing through a port to proxy the volume and rate of commercial activities in a certain area. Another interesting example is the use of images of shadow lengths from a real estate projects' construction sites to determine the project's pace.
Another popular source of alternative data is the sentiment of news and social media. Various applications of sentiment in finance and investing, such as systemic risk monitoring, stock selection, sector rotation and others, have shown that sentiment can be a powerful tool in risk reduction and in enhancing investor returns.
The value of an alternative data set
The usefulness of alternative data such as sentiment comes from its ability to instantly capture new price-moving information, providing an information edge to asset managers that use such data. Other alternative data sources can provide information that was recently unavailable. But how to assign the monetary value to an alternative dataset?
This question is interesting for data users as well as data providers, such as our partner Refinitiv. Information about how valuable a particular dataset is for investors, allows data providers to optimize their pricing schemes and increase revenues. On the other hand, the value of alternative data for data consumers (such as asset managers) lies in its monetizing potential and in increasing their returns.
The value of alternative data depends on the investor's profile and the fund's size. For example, signals extracted from some alternative datasets require immediate action, such as social media sentiment. So the value of such a dataset could be greater for investors who rebalance their portfolios more frequently. The size of a fund also plays an important role: a seemingly expensive dataset might generate many multiples of its cost in additional profits for a fund large enough – but possibly not for a smaller fund.
Another issue is that the value of alternative data depends on how it is going to be used. In other words: what are the signals extracted from these data and what is the envisioned investment strategy? Finally, the value of alternative data is not an absolute number, but it is always relative to a certain benchmark – which can be passive, such an index, or active, such as an alternative strategy (for instance, the one currently employed by the fund), which does not use such data.
Backtesting for assessing the value of data
The value of alternative data can be assessed from a data consumer's perspective through backtesting: by learning how an investment strategy would have performed had it incorporated alternative data in the past. The additional financial return – or alpha, the excess return over the agreed benchmark – can be used as the measure of a dataset’s value.
However, it is not the only performance measure used in investments. Risk is equally important in determining the performance of an investment strategy and alternative data can help reduce the risk of investments rather than directly enhance returns. So it is important to combine both these measures.
As the risk of strategy that uses alternative data might be quite different from the benchmark strategy, we need to make them comparable. For that, the benchmark can be leveraged or deleveraged to match its volatility to the volatility of the alternative data-driven strategy, making them comparable in terms of return.
Returns versus costs
The last step is to translate the overperformance into financial terms. For that, the additional return of the strategy (over the risk-adjusted benchmark) is multiplied by the fund's AUM. The resulting monetary value is the expected additional yearly income resulting from the use of the alternative data.
If this expected annual increase is significantly higher than the cost of purchasing, processing and onboarding the alternative dataset, then the asset manager can decide that it is profitable to buy and use this dataset in the investment process. Typically, the asset manager would calculate the multiplier – the recovery factor – which measures how many times over the dataset will earn its costs.
In our recent paper, soon to be published by Refinitiv, we illustrated the steps of this framework – from the choice of the investment strategy to its backtesting and to the valuation of the data – on the example of the alternative dataset of Refinitiv news sentiment. This example shows that such a dataset can earn back many multiples of its cost, even when compared with the active multifactor strategy, rather than a passive benchmark.
To conclude, we have seen time and time again how asset managers can be shocked by the price of a ‘sexy’ alternative dataset, quoted by a data provider. Instead, they should focus on what such a dataset can do for them, and our valuation framework will help them to do just that.
The full paper about the value of alternative data can be accessed on our Probability & Partners website: https://probability.nl/en/research-value-of-alternative-data/
Probability & Partners is a Risk Advisory Firm offering integrated risk management and quantitative modelling solutions to the financial sector and data-driven enterprises.