La Française: Machine learning-based currency risk management
La Française: Machine learning-based currency risk management
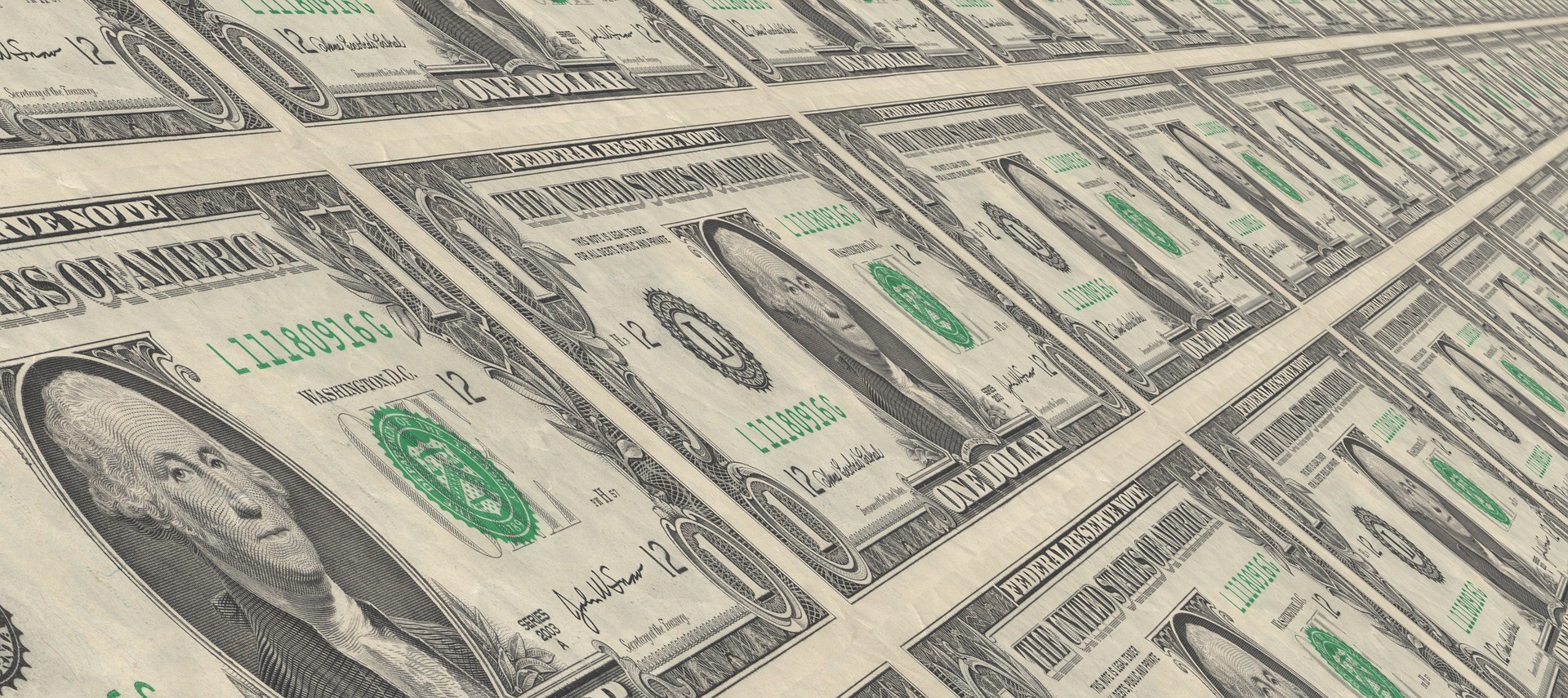
Diversification is said to be the only 'free-lunch' available on financial markets. The advancement of globalisation and free capital flows have enabled investors to spread their investments over different geographical regions, utilizing their less than perfect correlations. While such exposure partially reduces portfolio risk, it introduces a new component to the allocation: currency risk.
Currency risk, or fluctuations in foreign exchange, can contribute significantly to portfolio volatility, i.e. portfolio risk, as measured in the investor’s home currency, not only depends on fluctuations in foreign asset prices but also on variations in the associated foreign currencies. The latter is often more difficult to navigate, given the complexity of evaluating the risk of one currency against another.
Neglecting currency risk can lead to a suboptimal use of the risk budget and ultimately to the risk-reward ratio being negatively impacted by undesired currency fluctuations.
Currency overlay: the breadth of possibilities
To mitigate the negative effects of Forex exposure, investors can adopt a variety of approaches, the simplest being a passive hedge, which negates the foreign currency risk almost completely.
However, given the interest rate differential (IRD), the passive hedge is often the costliest approach to follow for the EUR-based investor. Given the cash requirements of futures and forward contracts, a static hedge tends to increase the liquidity risk of the portfolio.
Hence, investors can be forced to sell assets under unfavourable terms (i.e., consider the high cash liabilities from a USD hedge (ca. 16%) during the 2022 inflation-induced market decline). Under such circumstances, a static hedge would have interfered with taking advantage of the USD appreciation, eliminating all the benefits of what is widely considered a safe-haven currency in times of crisis.
Given ever-changing market conditions, the correlations between asset classes and currencies are dynamic, and the risk reducing characteristic of some currencies, such as the USD or JPY, varies. Given these limitations, investors often resort to a compromise, lying midway between a full static hedge and an unhedged investment strategy, the so-called: partial passive hedge.
In such a setting, the optimal hedging quote depends on several factors, including: hedging costs, portfolio cash flows, asset liquidity, diversification effects, regulations and market expectations. Although a partial hedge could be a good compromise, it remains a suboptimal solution due to the variability of the above-mentioned decision factors.
High costs also apply to the utilization of options, given their high upfront option premia. Furthermore, and as with any forward/future overlay strategy, investors require the expertise and an operational setup to efficiently implement and monitor such strategies, including regular hedge adjustments to the value changes in the portfolio.
The golden standard is consequently a systematic dynamic strategy which provides effective protection in the event of currency depreciation and likewise profit-taking in the event of currency appreciation.
Systematic dynamic hedging: the optimal strategy
The relationship between economic variables can be inherently non-linear. This is especially the case for the currency market, which is subject to a multitude of macroeconomic and political forces. Resorting to more simplified (often linear) models does not capture the complexity of interacting variables. The optimal hedging strategy is thus a quest to identify the appropriate methods to evaluate all the input variables as well as their interactions.
Consequently, the currency overlay strategy developed by La Française Systematic AM applies state-of-the-art supervised machine learning methods to tackle these challenges and derive an optimal hedging exposure. The machine learning approach is combined with a component based on behavioural finance and follows a model pooling approach, highlighting the fact that different models produce more accurate results in different market scenarios. While the system is recalibrated regularly, each model is selected based on forecasting accuracy and model stability.
For the calibration of the models, La Française Systematic AM’s currency overlay strategy makes extensive use of a large breadth of input data: macroeconomic, fundamental, cross-asset, momentum and sentiment.
Hence, the training of the supervised machine learning algorithm does not rely solely on simple and low 'signal to noise ratio' price data. At the same time, it demystifies the black box character of the machine learning model, as it is the quant researcher who influences the hedging characteristics of the strategy by selecting the relevant input data and by identifying the high-risk periods in the model’s training phase. The machine learning algorithms learn and select the appropriate settings and parameter values to identify such high-risk periods in the future.