Probability & Partners: This is how you get a grip on data quality under the new pension system
Probability & Partners: This is how you get a grip on data quality under the new pension system
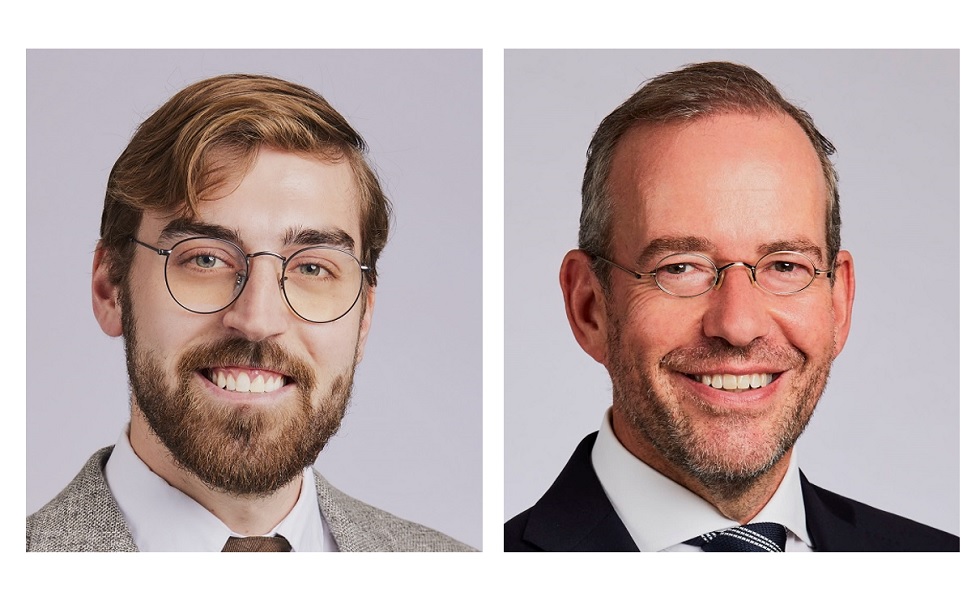
This column was originally written in Dutch. This is an English translation.
By Maurits van den Oever and Ronald Sijsenaar, Junior Risk Management Consultant and Partner at Probability & Partners respectively
Data quality is a significant point of attention for the pension transition. If the data registration is not in order, it is impossible to correctly determine how the collective buffers should be distributed among all participants. However, determining the risks and current state of data quality is a major job that involves multiple phases, perspectives and pitfalls.
The Pension Federation has published the Data Quality Framework to help with implementation. According to the Pension Federation, the pension provider who goes through this framework will have 'demonstrable insight into the data quality and can form an opinion about the data quality required to be able to enter'. The framework outlines a route of six phases that are iteratively connected. In order, the phases concern establishing the data quality policy and mapping the data elements, the risk inventory, the actual analyses, reporting, work by the external accountant and the final decision for entry.
The Data Quality Framework contains three concepts that play a central role in the data quality design and risk inventory and assessment. In order of appearance they are:
- KDE: Critical Data Elements.
- DRI: Participant Risk Indicator, an event in a participant's life that could have consequences for the KDEs.
- MTA: Maximum Allowable Deviation in the pension benefit.
Participant risk indicators and critical data elements
Phase 2, the risk inventory and assessment, requires the most thinking from the fund. An inventory must be made of the risk of data quality based on characteristics of the pension provider and characteristics of the participants. During the risk assessment of the participants, risk groups are determined based on their DRIs.
The chance of errors in the data is therefore inventoried on the basis of the DRIs. An important link is missing here in the Data Quality Framework. A DRI only becomes risky if there is a material impact on the claim. The interaction between DRIs and KDEs is not discussed in the Framework, while in our view this is an important nuance in the formation of risk groups.
There are different approaches to determining risk arising from a DRI. After all, errors can occur in several places in the data flow. First of all, errors can come from the source of data. These are mainly the DRIs where the change of data is initiated or entered manually. An example of this is the DRI 'Separation/Settlement/Conversion'. If a participant divorces, agreements must be made about the division of the pension. According to the Data Quality Framework, the source of the pension distribution is the participant or the participant's ex-partner. Errors can therefore be made when passing on this data.
The way data is processed can also introduce risks. Most changes will be automatic. If this is the case, the chance of errors is nil. If this is not the case, more attention will have to be paid to checking the changes. This can therefore also be taken into account when assessing risks. A DRI where data is mainly processed manually and the checks are not watertight, may therefore be a reason to place participants with this DRI in a risk group.
As previously noted, a DRI will only be risky if it has an impact on the claim. Not every DRI is the same in this regard. Any DRI that has a direct effect on the calculation parameters of the entitlement, such as the 'Flexibilization of Pension Benefit', is a clear candidate for having a material impact. DRIs that mainly affect personal data such as 'Marriage' require less attention here.
Maximum Allowable Deviation
After identifying and assessing the risks, a Maximum Allowable Deviation must also be determined at the end of phase 2. In essence, this is the quantitative measure of your risk appetite. The amount of the MTA is determined based on the risk assessment. This could imply that the MTA per participant is lower for small funds. Because more data processes are manual, you want to look at your data risk more prudently, and therefore accept fewer errors. The established MTA also has implications for your operational reserve. If the MTA is set low, less money will have to be set aside for possible claims.
After the MTA has been determined, another issue will arise. How do you deal practically with your MTA? It is difficult to assess for each individual participant what the deviation is and then not correct it if the deviation is smaller than the MTA. Realistically, a hybrid form of assessment is the solution for this. For all participants who do not explicitly belong to a risk group, random checks can be made to see whether there are many observations that exceed the MTA. The risk groups will have to be examined in more detail. This can be done either by including more observations in the sample, or by simply including every participant in the risk group in the data analysis.
Conclusion
The Data Quality Framework is a comprehensive route description for the 'Get clean, stay clean' principle of data quality the WTP and provides valuable tools for managing data quality risk. In addition to the Framework, we propose to look at establishing a link between DRIs and KDEs and creating a risk ranking, and analyzing the correlation of complaints and incidents with the KDEs. It is also relevant to establish a connection between the risk appetite, the MTA, and the operational reserve. We also propose to set up data quality controls taking into account the risk ranking of the DRIs.