Svetlana Borovkova: Fairness in AI and machine learning
Svetlana Borovkova: Fairness in AI and machine learning
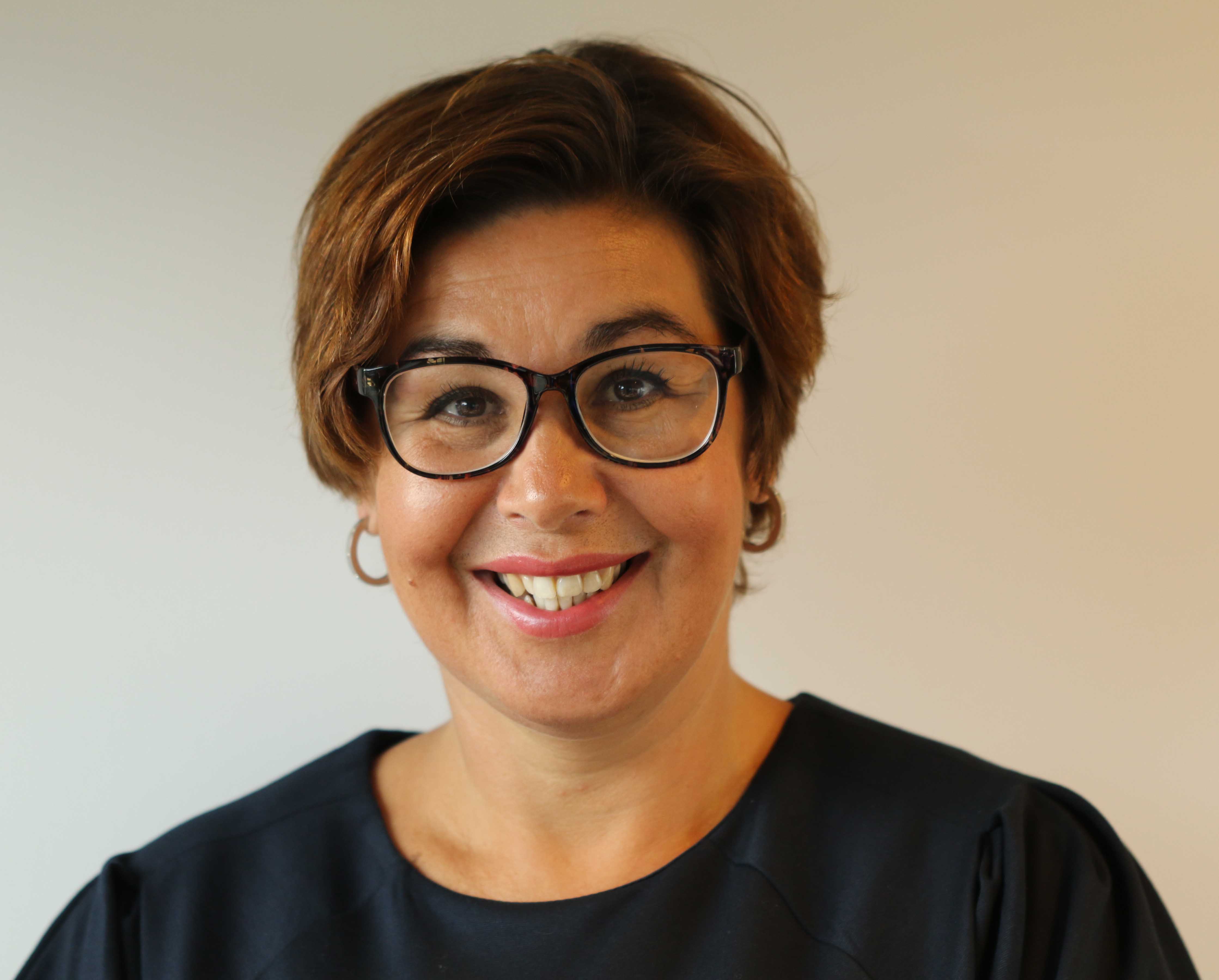
By Svetlana Borovkova, Head of Quant Modelling at Probability & Partners
Artificial Intelligence (AI), powered by Machine Learning algorithms, is finding its way into the financial system. AI is currently applied in financial institutions in immaterial areas such as marketing (product recommendation) or customer service (chat bots). However, slowly but surely AI is gaining ground in high-stakes decision making such as credit issuance, insurance underwriting and identification of fraud and money laundering.
These applications are potentially very sensitive to unfair treatment of different groups or individuals. Such an unfair, biased treatment is particularly damaging for finance sector, as it is held in higher societal standards than other industries, because it is fundamentally based on trust.
Machine Learning algorithms learn patterns from historical data, which they then carry forward. So any potential bias reflected in that past data will be reflected – and possibly amplified – in the outcomes of a ML algorithm.
One famous example is the Apple/Goldman Sachs credit card, unveiled to a great fanfare at the end of 2019. Apple proudly declared that the credit limit on their card will be determined solely by an AI algorithm, only to find out, a few days later, that women got on average 10 times less credit than men, even in cases of the same or higher credit score. One can only imagine the reputational damage this has inflicted on Apple and especially on Goldman Sachs.
Avoiding such disparate treatment of certain groups of society – based on gender, race or age – is at the heart of AI fairness. At the end of 2019, the ECB came up with Ethics Guidelines for Trustworthy AI. The Dutch Central Bank has followed the suit, by issuing the so-called General Principles for the use of AI in the financial sector. Two out of six of these principles – Fairness and Ethics – are directly related to fair and unbiased application of AI.
Whether an AI or ML-aided algorithm is fair can – and should be – assessed before this algorithm is adopted in practice, and potential bias of an algorithm can be quantitatively measured. But for that, first we have to define the so-called protected attributes. In other words, those features of an individual on basis of which a person should not be discriminated. That also means he or she mustn’t denied credit or an insurance policy.
Typical examples of protected attributes are race, gender, age, sexual orientation or religion. Protected attributes are determined by law, but financial institutions can outline their own ethical standards (alongside those enforced by regulation) and ensure their AI algorithms comply with those standards as well.
The main risk resulting from AI algorithms being unfair is reputational risk, and it is particularly damaging for financial institutions. So ensuring fairness of your AI solutions should be one of the routine tasks of risk managers and all those responsible for implementing AI and ML in your organization.
In my next blog, I will discuss, in simple terms, how bias in ML applications can be measured and mitigated. Until then, I invite you to think of your organization’s ethical standards you would like to see your AI algorithms adhere to.
Probability & Partners is a Risk Advisory Firm offering integrated risk management and quantitative modelling solutions to the financial sector and data-driven enterprises.