Svetlana Borovkova: Large Language Models in Finance and Investing
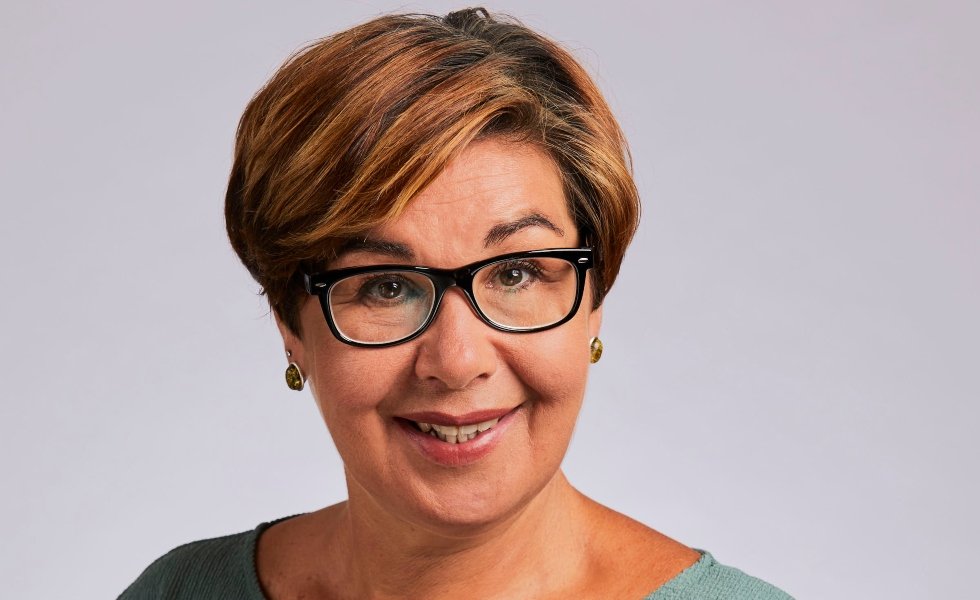
By Dr. Svetlana Borovkova, Head of Quant Modelling at Probability & Partners
Generative AI and LLMs are the most significant technological developments of the past few years. Since their emergence less than two years ago, these tools remain the talk of the day, also in financial institutions.
As these tools find their way into our ways of working, we are gaining a deeper understanding of their capabilities. In financial services, professionals and quants are identifying areas where LLMs can be applied, such as document summarization, code writing and many others. However, there are still, often uninformed, assumptions that LLMs (large language models) will replace many finance professionals. With this column I hope to highlight some of pitfalls of using LLMs in finance and investment and in my next column I will go into more detail about how to mitigate them.
Current LLM applications
An LLM is a machine learning (ML) model – a neural network – trained to interpret and generate human-like text in a wide range of contexts. These models have an enormous number of parameters (weights). For example, ChatGPT 3.5 has 175 billion of them and they are trained on very large textual datasets, involving, for example, the entire corpora of Wikipedia. As a result, LLMs are able to handle a wide variety of tasks, ranging from writing poetry to improving and (re)writing text in practically any style or context. This is LLMs’ main strength, but herein also lies their fundamental weakness: the lack of domain-specific knowledge, often needed in precise finance-related tasks.
For example, a recent study by Alexandria Technology shows that ChatGPT is less able to interpret financial analysts’ calls than proprietary natural language processing (NLP) models, specifically tailored to this task. Also, a recent study by LSEG (London Stock Exchange Group) shows that the analysis of financial news by ChatGPT is inferior to smaller NLP and ML models, trained on domain-specific data, such as other financial news.
Currently, LLMs are seen as a ‘co-pilot’ for finance professionals. Their main advantage lies in relieving humans of tedious, repetitive, and time-consuming tasks. For example, LLMs are good at summarization of and information retrieval from lengthy and verbose documents such as analysts’ reports, financial contracts or loan offerings. These contracts also can be checked by LLMs for compliance with existing regulations. However, to effectively generate – rather than summarize – such documents, LLMs need to be fine-tuned on a large database of similar documents.
Many other applications of LLMs are currently emerging, all of them geared towards efficiency or improved customer experience. For example, a recently unveiled tool by Salesforce, Einstein GPT, powered by the OpenAI technology, is an LLM fine-tuned to Customer Relation Management applications.
Peeking into the future
Looking ahead, more exciting applications of LLMs can be envisaged, such as sentiment analysis of financial news, blogs and other finance-related communications or risk analysis and development of early warning risk systems. However, for such applications LLMs should be adapted to these tasks by re-training or fine-tuning them on domain-specific data, allowing LLMs to acquire much needed specialized knowledge. In my next article, I will go into more detail of how an open-source LLMs (such as Llama) can be fine-tuned with a limited computing power and cost – techniques for this, such as LoRA and QLoRA are rapidly emerging.
If we look even further into the future, we might be tempted to fantasize about highly anticipated applications such as creating profitable investment strategies and generating alpha. In my view, such applications are more distant than one might wish. There are several reasons for this. First of all, such applications involve generating true intelligence, while LLMs are designed for language generation rather than abstract reasoning. Because LLMs sound so ‘intelligent’, people naturally assume they really are.
There is another fundamental obstacle to using LLMs for quant investment and trading strategies: the impossibility of backtesting, while ensuring careful elimination of any forward-looking bias. This is impossible with LLMs. All foundational LLMs are trained on huge textual databases up to a specific date (for example January 2022 for ChatGPT 3.5 and April 2023 for ChatGPT 4), and then their weights are frozen.
However, for backtesting of a strategy constructed with the help of an LLM, we need the state of this LLM at each point in time in the past, like an LLM trained on the basis of information available up to that point in time but not further. As this is not the standard training process of an LLM, it will always have a forward-looking bias which cannot be eliminated. Consequently, this can lead to investment strategies appearing more profitable than they really are.
Other issues and solutions
There are other pitfalls of LLMs, some are more well-known than others, and tools are emerging to deal with them. One, for example, is timeliness: if an LLM is trained on the data up to, say, April 2023, it will not ‘know’ anything that happened after that date. Another famous pitfall are the so-called hallucinations: generating plausibly sounding but factually incorrect answers. Both of these issues can be dealt with by combining an LLM with the access to the Internet or an external database: the technique called Retrieval-Augmented Generation (RAG).
Another potential pitfall in using LLMs in financial services is the issue of explainability, fairness and compliance with the current regulation such as the EU AI act. These issues are harder to deal with than, say, hallucinations, but still deserve attention. Another controversial topic is the sustainability and electricity consumption needed for training foundational LLMs. It is estimated that this electricity need already exceeds that for Bitcoin mining and will amount to 12-13% of global electricity production in the next couple of years. So ‘green’ ways of dealing with this problem are needed.
Finance professionals, especially at the top level, must be aware of both opportunities and limitation of LLMS when designing a financial institution’s AI policy, and it is my hope that this piece is a step in this direction. Fortunately, a myriad of ways of dealing with many of these problems are emerging. These will be highlighted in my next column.
Probability & Partners is a Risk Advisory Firm offering integrated risk management and quantitative modelling solutions to the financial sector and data-driven enterprises.