Svetlana Borovkova: Machine learning for credit
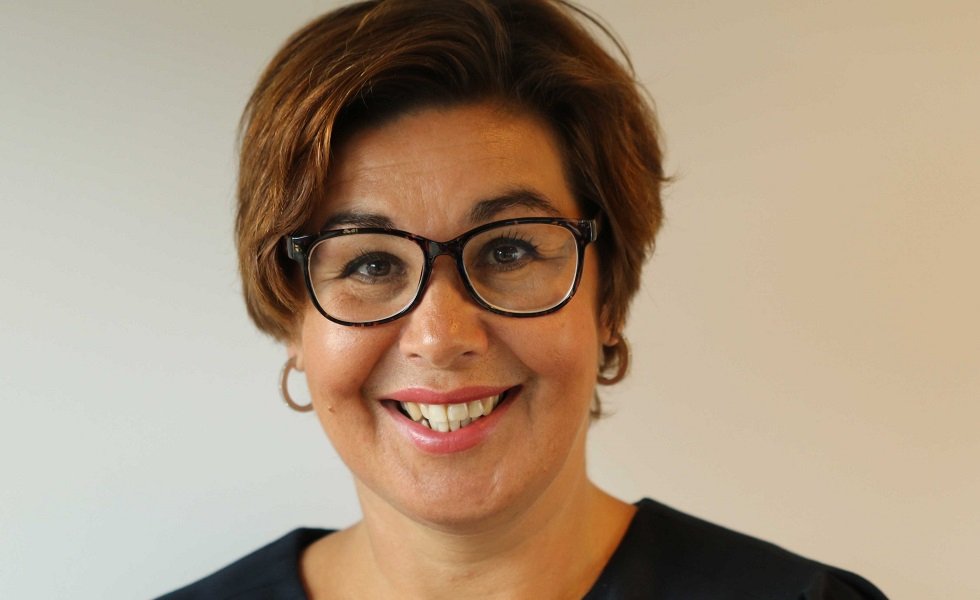
By Svetlana Borovkova, Head of Quant Modelling at Probability & Partners
On November 11th 2021, The European Banking Authority published a discussion paper on Machine Learning for an Internal Rating-Based approach. This paper is the first serious step towards the acceptance of using machine learning models for credit applications.
In recent years, there has been much interest from financial institutions in exploring machine learning models for credit-related topics, ranging from assessing the creditworthiness of new credit applicants to monitoring existing loans and flagging those that are potentially ‘troublesome’.
For asset managers, these new developments can also be interesting: in search for yield, more and more asset managers invest in credit portfolios (or mortgages, SME, or other loans) and for them, machine learning models can be beneficial for estimating losses of such portfolios.
However, scepticism about such models has been quite significant. Applying machine learning in the area of credit has many potential pitfalls, which we will summarize here.
Interpretability of ML model outcomes
One such pitfall – and the one most often voiced by sceptics – is seeing machine learning models as a kind of ‘black box’ because of the perceived inability to interpret the model’s outcomes.
This criticism of machine learning models is quite outdated and the easiest to refute. There are several advanced methods, specifically developed for machine learning algorithms, that allow for a comprehensive interpretation of the model’s structure and outcomes, on a global as well as on local levels. These methods are for instance SHAP (Shapely Explanation) and LIME (Local Interpretable Model-agnostic Explanations), partial dependence plots and counterfactual explanations, among others.
Biases and fairness of ML applications
Another pitfall, especially relevant for credit issuing institutions, is the substantial scope for undesirable biases and lack of fairness in ML model outcomes. This can be a huge reputational risk and banks applying ML models for credit must be acutely aware of these potential biases. The reason for such biases lies in the perpetuation (and even amplification) of historical biases in credit – something machine learning algorithms are particularly prone to as they are very good at recognizing patterns in historical data and carrying them forward in their predictions.
Fortunately, there are many bias detection and mitigation tools available, for example, IBM AI Fairness 360 toolbox or FairML toolkit available on GitHub. It is important that quants and model developers are adequately aware and skilled in recognizing and measuring these biases and familiar with the available mitigation tools.
Human involvement: still much needed
The next potential pitfall in ML applications is the perceived lack of human involvement. A common misconception is that you can just throw a whole lot of data at a machine learning algorithm and it will provide you with an answer.
Nothing could be further from the truth. Human intervention and involvement (by a modeller, data analyst or another stakeholder) is essential at several stages of ML model development and application, starting from data collection and data pre-processing, to feature selection and hyperparameter tuning and finally to explaining the outcomes to other stakeholders and clients.
Privacy and GDPR
Other issues are data integrity, privacy and GDPR. These are more forward looking rather than imminent. Currently, ML credit models are typically trained on the basis of the same kind of data as the existing credit models. In such situations, the data has been collected and used for the purpose of credit issuance and monitoring and hence there is no fundamental difference between using these data in current or ML models.
The situation can potentially change dramatically in the future, when new, alternative datasets might be used to assess creditworthiness of applicants, such as spending patterns on credit/debit cards, browsing history or content posted on social networks. This indeed has a huge potential for privacy issues and GDPR compliance, so financial institutions that are thinking of using alternative data in their ML models should be acutely aware of this.
Some technical pitfalls
There are also some more technical – but nevertheless just as important – issues that ML models can run into. One example is the tuning of hyperparameters of a ML model (those parameters that have to be chosen beforehand rather than estimated from the data).
Another example is the fact that many ML algorithms continue ‘learning’ (adjusting and refitting themselves) even after the formal training process is complete: that is during the production/deployment phase. Examples of such algorithms are reinforcement learning algorithms, among others. In such situations, the models can ‘run away’ from you and the initially trained model that you thought was well-assessed and validated can become a different model after a while, both in its structure and its outcomes.
Model governance and validation
But the two most prominent issues with the use of machine learning models are more human- and operational-related. The first is the lack of skill and expertise within financial organizations to understand and deploy machine learning models. The second is the need for complete re-adjustment of your model governance landscape, to include the use of machine learning models. Here one should think of issues such as model ownership and accountability or challenges related to ML model validation.
One thing is certain: the recent EBA discussion paper is an important first step towards regulatory acceptance of ML models, which can provide significant benefits for banks and asset managers. Financial institutions should start preparing to incorporate these models into their modelling landscape if they do not want to fall behind.
Probability & Partners is a Risk Advisory Firm offering integrated risk management and quantitative modelling solutions to the financial sector and data-driven enterprises.