Svetlana Borovkova: Challenges and pitfalls of Economic Scenario Generation
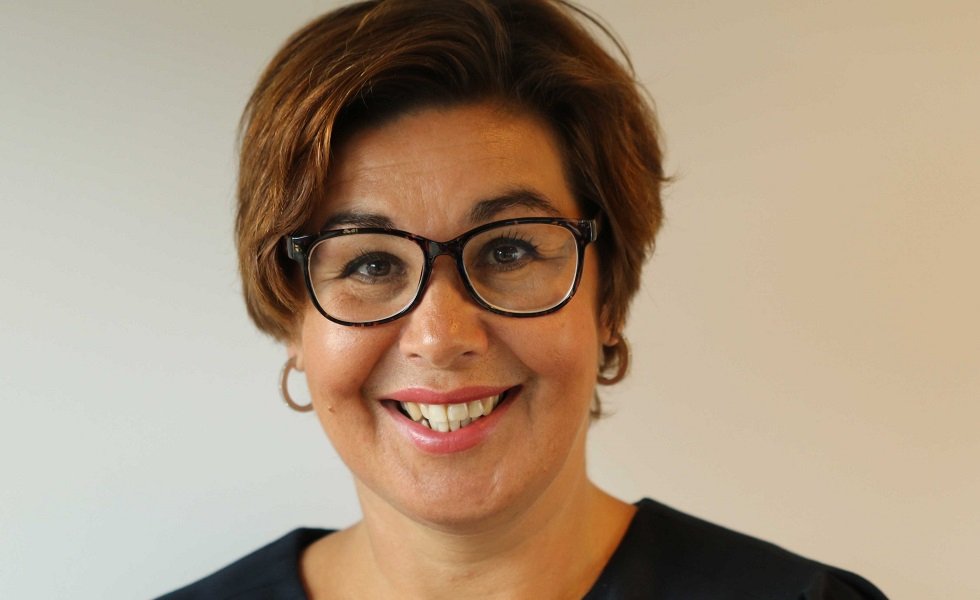
By Svetlana Borovkova, Head of Quant Modelling at Probability & Partners
Economic Scenario Generators are tools that simulate future paths of economic, financial and market variables. They are essential to many applications, for instance in investment and asset management. These generators evaluate the performance of investment portfolios under a multitude of plausible economic scenarios and reveal potential risks.
The Economic Scenario Generators used in asset management must provide future simultaneous paths of interest rates, equity markets and possibly other asset classes (high-yield investments, commodities and others). Time horizons for the required simulated paths can be quite long. For pension funds, for instance, these must extend 30 years or more into the future.
Outcomes from these generators need to cover the full range of future scenarios. They must give realistic distributions of financial variables (for instance interest rates or equity returns) at the required (possibly long) time horizon. This means that unlikely but plausible outcomes also need to feature in the scenario set, as these are typically responsible for tail risks.
The risk of overestimating implausible risks
And herein lies the first major pitfall of creating a good Economic Scenario Generator. In the quest to cover these unlikely but risky scenarios, analysts often lose track of what is plausible, and their generators come up with scenarios that are undoubtedly very risky, but also completely unrealistic from economic and historical perspectives.
For example, I have seen generators producing interest rates of -5% or significantly negative (aggregated) equity returns over a 30-year horizon. Undoubtedly, these would be very risky situations, but are they plausible? In my opinion they are not. So, there should always be some kind of ‘sanity check’ of the outcomes, to avoid sacrificing common sense for the sake of (extremely) prudent risk estimates.
The pitfall of having an unrealistically large spread of future values
Another, related pitfall is an unrealistically large spread of future values (for example interest rates or equity returns) at long horizons. In a widely used equity model – the so-called Geometric Brownian Motion – the volatility of future values increases indefinitely, as the square root of time.
Although mathematically appealing, this is completely unrealistic. An alternative model, called Mean Reversion, where interest rates, commodity prices or equity returns tend to return to their long-term means, offers an elegant solution to this problem. In such a model, the volatility stabilizes around some bounded value, even for long time horizons.
The dangers if mechanically applying the Mean Reversion Model
However, mechanically applying this model is also not without dangers. If this ‘long term mean’ is assumed to be constant (for example equal to the long-term average of interest rates), then, given the current negative rates, the model will estimate the expected time to revert to the long-term average of, say 2% or 4%, as being nearly infinite. In this case, the analyst, with his hands in his hair, will blame the estimation procedure, lack of data or any other factor for these runaway estimation results.
But the simple reason for this is the fact that the ‘long-term mean’, or the ‘equilibrium level’ of rates, returns or prices is not constant. It also changes in time, albeit much more slowly than the financial variables themselves.
For example, it would be silly to insist that the ‘mean oil price’ is, say, 50 $/bbl at times when oil was over 140 $/bbl (such as in 2008). Consequently, fitting a Mean Reversion model with that fixed mean level is akin to banging a square peg into a round hole, and the estimates of other model parameters are bound to be unrealistic in this case.
Incorporating a slowly varying mean into mean reversion models immediately solves all these identification issues and can be done with the powerful technique of Kalman filtering, known from signal analysis. Economic scenario generators can then produce the future paths of financial variables, also displaying these slowly varying mean levels (which in turn can reflect the economic cycle) and illuminate the additional uncertainties associated with them.
Further challenges
Another major challenge when designing a good Economic Scenario Generator is a realistic modelling of the complex (and changing) relationships between financial variables, such as interest rates and equity returns. But this is a separate topic, which I will address in my next column.
I would like to conclude with a famous saying by a Danish Nobel prize-winning physicist Niels Bohr: ‘Prediction is very difficult, especially when it is about the future!’ Economic scenario generation is more of an art than a science, which requires experience, economic insights and intuition, alongside advanced quantitative skills.'
Probability & Partners is a Risk Advisory Firm offering integrated risk management and quantitative modelling solutions to the financial sector and data-driven enterprises.